Luis Martín Mejía-Mendoza, Martha M. Flores-Leonar, Andrés Aguilar-Granda, Jose D. Perea-Ospina, and Alán Aspuru-Guzik
We had a recent publication in Current Opinion in Green and Sustainable Chemistry that summarizes what a materials acceleration platform is. In this Substack post, we want to share our excitement for MAPs with our community of readers. We refer them to the article (for which you can request a copy here by finding the publication and clicking on Request PDF) for more references and detailed discussions.
Materials Acceleration Platforms (MAPs), the new paradigm to accelerate materials discovery are an effort to develop technology solutions that can help alleviate climate change concerns. A MAP, conceived as a self-driving laboratory (SLD), combines robotic platforms with artificial intelligence (AI) to achieve autonomous experimentation for clean energy materials. We identify five key components in a MAP: AI models, robotic platforms, orchestration software, storage databases, and human intuition. These components are interconnected and integrated under a closed-loop approach, replacing the traditional paradigm of design, synthesis, characterization, and testing.
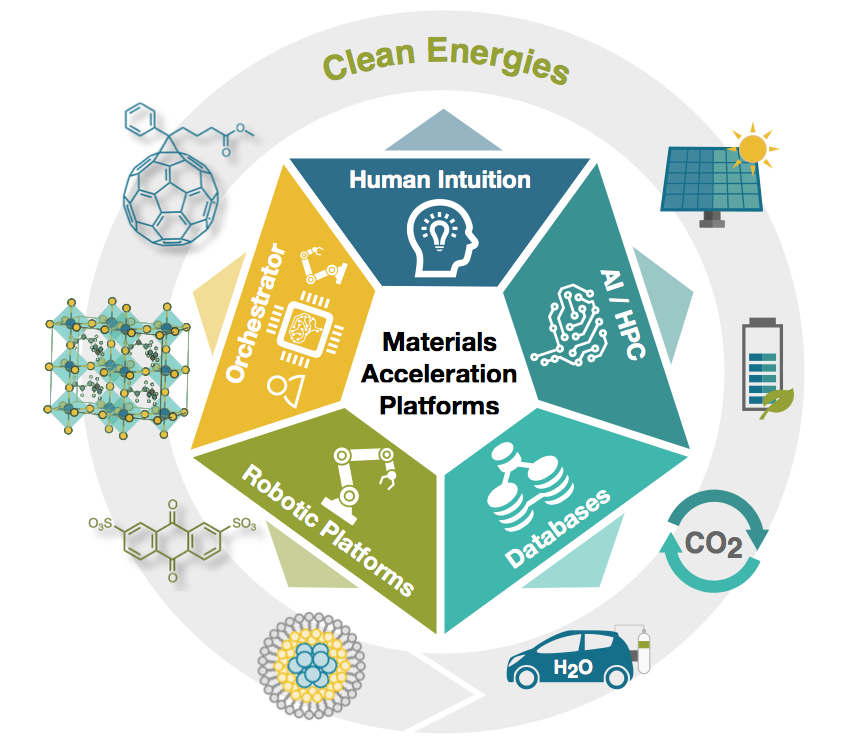
On the path to autonomous experimentation, we can find a variety of attempts, including robotic platforms, machine learning models, and integrated MAPs.
Historically, the automated synthetic processes and robots have their origins in peptide synthesis and the pharmaceutical industry. The automation of modern chemistry offers benefits, for instance, increased speed and throughput, higher reproducibility, low consumption for materials, and, consequently, the possibility of a more comprehensive exploration of the chemical space. Nevertheless, the current automated platforms tend to be limited by reaction type and experimental conditions, such as temperature, concentration, and solvent.
As an example, a promising approach to the automatic generation of small molecules has been developed in Burke's laboratory. This approach combines both a flow and a batch setup to perform C-C couplings using a three-step reaction. A relevant feature is the in-line catch-and-release purification protocol developed for this molecular building block approach. Another configuration of synthesis platforms involves a plug-and-play modular design and miniaturized microfluidic synthetic devices, which play a central role in the generation of small molecules through diverse chemical transformations. Although most of these systems are efficient, they remain expensive in terms of time and resources. Artificial intelligence methods can enhance platforms to accelerate the pace of materials discovery and reduce its cost.
Artificial intelligence (AI) technologies play a crucial role in MAPs thanks to their ability to improve automatically through experience (data). Inverse design and active learning are two significant areas that are worth attention. As mentioned above, robotic platforms provide high volume, control, and precision over experimental processes; they can generate high-quality data. One example where active learning is applied is the constrained (e.g. time, budget, feasibility) optimization of high-dimensional parameter spaces (e.g. reaction conditions, material properties). In this task, our team has developed different bayesian optimizers for continuous and categorical variables. On the other hand, the inverse design seeks to generate candidate materials with desired properties, complementing the "idea generation" component of MAPs. Generative models, such as variational autoencoders, generative adversarial networks, and hybrid methods, offer an efficient way to explore chemical space, estimated in ~1060 molecules.
Finally, a MAP requires the integration and management of robotic platforms, machine learning models, and users. For this purpose, orchestration software, such as ChemOS, has been developed for autonomous experimentation, providing accessible communication between components of a MAP for efficient experiment planning.
An example of SDL, driven by ChemOS, is the effective optimization in multicomponent experimentation materials discovery for organic solar cells' active layers. In this approach, new experiments are proposed based on all the measurements collected before for the performance of the photo-stability in the active layer. To this end, the ML decision-maker infers the results of all possible experiments, taking advantage of the statistical correlations identified from the previous measurements, and suggests the most informative ones for future evaluation.
Stay tuned for more news coming out about MAPS soon!
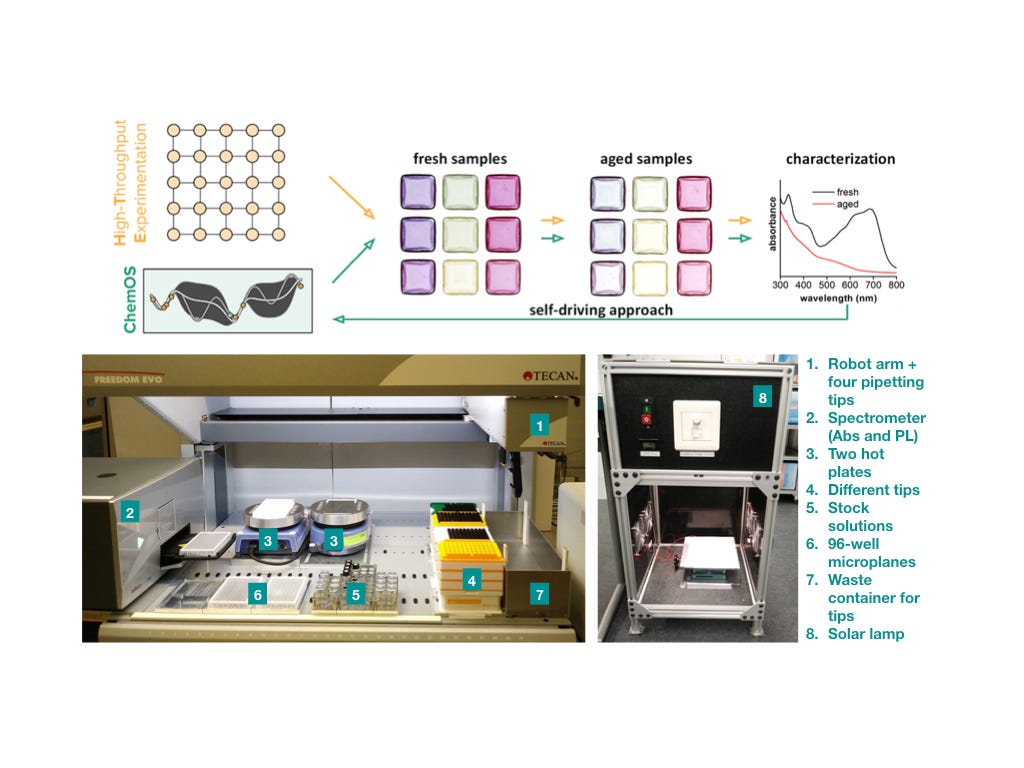